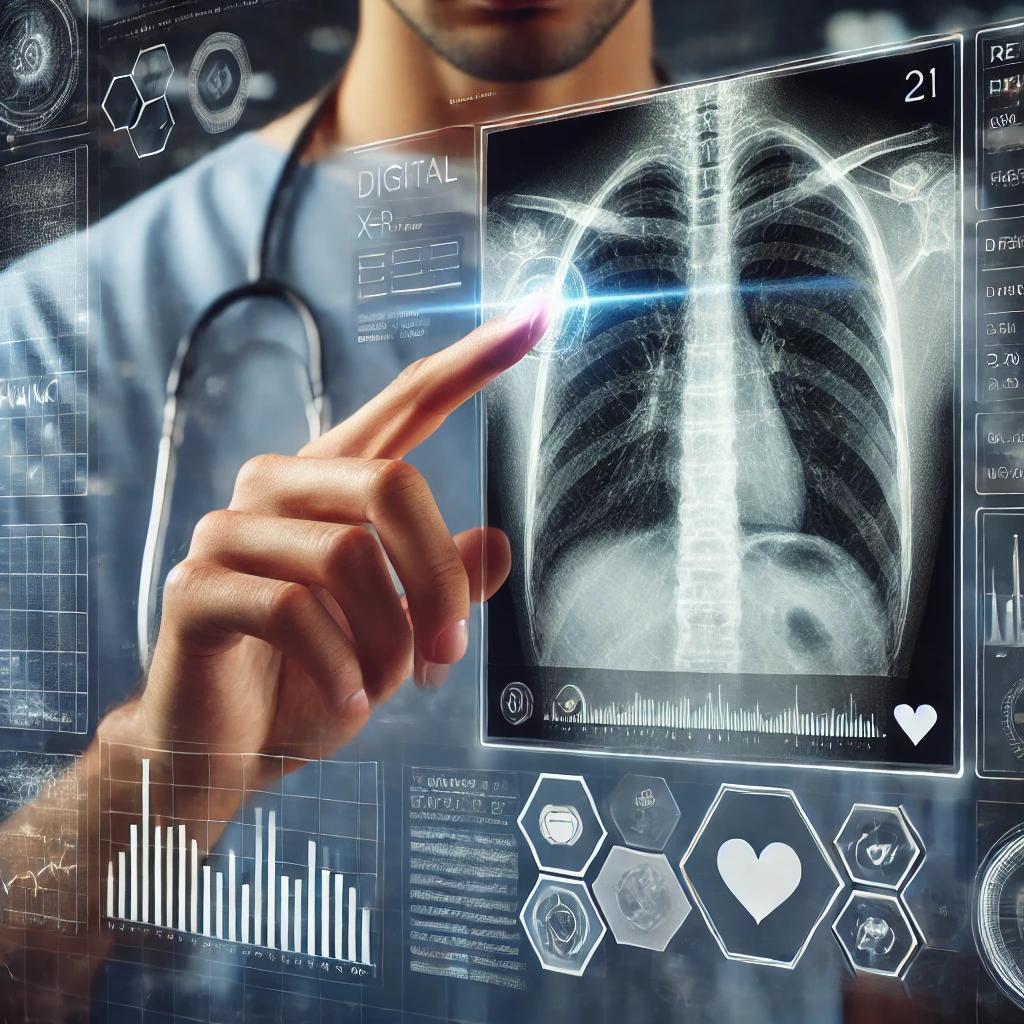
This article explores how AI technologies support medical imaging analysis, genetic profiling for precision medicine, and predictive analytics for early disease detection. It also delves into advancements in treatment approaches, including personalized care plans, robotic-assisted surgeries, and accelerated drug discovery.
AI in Healthcare
The healthcare sector has been undergoing rapid transformation with the integration of artificial intelligence (AI). Today, AI’s influence extends from hospital diagnostics to at-home patient monitoring, promising improvements in the speed, accuracy, and efficiency of medical care. According to a recent report by Accenture, the AI healthcare market is projected to reach $6.6 billion by 2024, driven by increasing demand for intelligent solutions that reduce operational costs and enhance patient care.
A primary reason for this shift is the vast data generated by healthcare systems, including electronic health records, imaging scans, genomic sequences, and wearable device metrics. AI algorithms process this data faster and more effectively than traditional methods, empowering healthcare professionals with actionable insights. This article delves into these transformative applications, from diagnostic imaging to personalized medicine, and explores the ethical considerations essential for sustainable AI adoption in healthcare.
Transforming Diagnostics with AI
One of the key ways AI is enhancing healthcare is through its application in diagnostics, where AI-driven tools help detect and interpret medical data. Advanced algorithms analyze various data types, from imaging to genetic sequences, identifying patterns and anomalies with precision that rivals – and often exceeds – human capabilities.
1. AI in Medical Imaging
AI’s ability to enhance medical imaging is one of its most celebrated applications in healthcare. Recent studies reveal that AI-assisted diagnostics can detect certain conditions, such as breast cancer, up to 30% more accurately than traditional human assessments. The process involves training AI algorithms on vast image datasets, allowing them to recognize patterns indicative of specific diseases.
- Lung Cancer Detection: AI-based systems have shown high accuracy in detecting early-stage lung cancer. Google Health’s deep learning model, for instance, demonstrated superior detection rates over human radiologists, identifying nodules in CT scans with fewer false negatives.
- Retinal Disease: In ophthalmology, AI has made significant strides in identifying retinal diseases like diabetic retinopathy. In India, the Aravind Eye Hospital uses AI-powered tools to screen millions of patients each year, improving early intervention and reducing blindness rates.
These applications improve diagnostic accuracy and reduce the workload for healthcare professionals, allowing them to focus on more complex patient care tasks.
2. Genetic Analysis and Precision Medicine
Genetic analysis has become more accessible and affordable, largely due to advances in AI. With machine learning algorithms analyzing genetic data, healthcare providers can now offer precision medicine, tailoring treatments to an individual’s genetic profile.
- Cancer Treatment Customization: AI-driven genomics helps identify mutations that respond well to specific cancer treatments. For example, Tempus, a precision medicine company, uses AI to analyze genetic data and suggest treatment options that maximize efficacy and minimize side effects.
- Rare Disease Detection: AI has been particularly useful in identifying rare diseases, which are often misdiagnosed due to their subtle and complex symptoms. Machine learning models analyze genetic markers to provide more accurate and timely diagnoses, offering life-changing insights for patients and families affected by these conditions.
3. Predictive Analytics for Early Disease Detection
AI’s predictive capabilities are invaluable in identifying individuals at risk for chronic diseases. By analyzing patient histories, lab results, and lifestyle factors, AI models predict disease development, enabling early intervention.
- Heart Disease Prediction: AI models analyze EHR data to identify patients at high risk for heart disease, often months or even years before symptoms arise. Studies show that predictive models increase early diagnosis rates, allowing preventive measures that could save lives.
- Diabetes Prevention: With predictive models, healthcare providers identify individuals who are prediabetic, enabling lifestyle interventions to prevent diabetes onset. Programs such as these are especially valuable in primary care, where early action can reduce long-term healthcare costs.
AI’s Role in Treatment Advancements
AI is instrumental in evolving medical treatments, making care more personalized, efficient, and effective.
1. Personalized Treatment Plans
Personalized medicine, also known as precision medicine, leverages AI to offer tailored treatment plans that maximize therapeutic success.
- Chemotherapy Personalization: For cancer patients, chemotherapy regimens are often challenging to optimize. AI algorithms analyze patient data to recommend individualized chemotherapy doses, reducing toxicity while enhancing treatment effectiveness.
- Chronic Disease Management: Personalized treatment plans for conditions like asthma and chronic obstructive pulmonary disease (COPD) are developed using patient health data and lifestyle metrics. AI-driven tools monitor symptoms, provide insights on medication adjustments, and encourage adherence to treatment protocols.
2. Robotics in Surgery
Robotic surgery, guided by AI, allows for increased precision in complex procedures. These robots can conduct minimally invasive surgeries, reduce the risk of human error, and decrease recovery times.
- Da Vinci Surgical System: The Da Vinci system, which combines robotic technology with AI guidance, enables surgeons to perform intricate operations with enhanced control. Used in urological, gynecological, and cardiac surgeries, this system has resulted in fewer complications and faster patient recovery.
- Orthopedic Surgeries: AI-guided robotic systems in orthopedic surgeries assist with joint replacements, offering precise alignment that minimizes post-operative complications and improves long-term outcomes.
3. Drug Discovery and Development
The drug discovery process is traditionally slow, requiring years of research and massive financial investment. AI accelerates this process by analyzing biological data to identify promising drug candidates and predicting drug efficacy with high accuracy.
- COVID-19 Drug Discovery: AI was instrumental in identifying potential compounds and accelerating COVID-19 vaccine development. IBM Watson and BenevolentAI leveraged machine learning to analyze existing drugs for repurposing, contributing to the rapid development of treatments.
- Antibiotic Discovery: MIT researchers developed an AI model to screen existing compounds for antibiotic properties, leading to the discovery of Halicin, a powerful antibiotic effective against antibiotic-resistant bacteria.
Ethical Considerations in Healthcare AI
The rapid adoption of AI in healthcare brings ethical concerns that must be addressed to ensure responsible use.
1. Patient Privacy
AI depends on vast amounts of patient data, including medical records, genetic information, and imaging data. Protecting this sensitive data is essential, as breaches could have severe consequences for patients’ personal lives and trust in healthcare systems.
- Data Encryption and Anonymization: Healthcare providers must implement robust encryption and anonymization techniques to protect patient data. Organizations like Health Level Seven International (HL7) develop standards to ensure secure data exchange in healthcare settings.
- Compliance with Regulations: Compliance with privacy laws, such as the Health Insurance Portability and Accountability Act (HIPAA) and General Data Protection Regulation (GDPR), is crucial for protecting patients’ rights.
2. Algorithmic Bias
Bias in AI algorithms can lead to unequal treatment outcomes. For instance, if an AI model is trained primarily on data from one demographic, it may fail to provide accurate results for other groups.
- Diverse Training Data: Ensuring diversity in AI training datasets is vital to minimize biases. Initiatives like the Algorithmic Justice League advocate for inclusive data collection and transparent AI development to avoid discriminatory outcomes.
- Regular Bias Audits: Conducting periodic audits of AI algorithms helps identify and address biases, fostering fair treatment across diverse patient populations.
3. Over-Reliance on AI
AI should be a supportive tool, not a replacement for human judgment in healthcare. Over-reliance on AI can lead to potential oversights in critical decision-making scenarios.
- Human-AI Collaboration: Effective use of AI requires a balanced approach, combining AI insights with healthcare professionals’ expertise. Collaborative AI systems are designed to provide recommendations while allowing human professionals to make final decisions.
Case Studies: AI in Action in Healthcare
Case Study 1: Early Detection of Breast Cancer with AI
AI has proven particularly beneficial in breast cancer screening. In a study conducted by MIT, AI-assisted systems demonstrated improved accuracy in detecting early-stage breast cancer compared to traditional mammogram reviews.
Case Study 2: AI-Assisted Diabetic Care in Primary Clinics
At Mayo Clinic, AI-driven applications monitor diabetes patients’ blood sugar levels, physical activity, and dietary habits to provide personalized recommendations. This real-time data support helps patients maintain optimal glycemic control, reducing long-term complications.
Case Study 3: Enhancing Mental Health Support Through AI Chatbots
AI chatbots, such as Woebot, engage in conversations with users to provide mental health support. These chatbots employ natural language processing to offer resources, strategies for stress management, and notifications to mental health professionals if a higher level of care is needed.
Case Study 4: AI in Sepsis Prediction
AI models at hospitals like Johns Hopkins University predict the onset of sepsis by analyzing patient data, enabling faster interventions. Sepsis prediction algorithms have demonstrated up to 85% accuracy, offering a critical tool for healthcare providers in intensive care units.
Case Study 5: Remote Patient Monitoring for Heart Disease
AI-powered wearable devices monitor patients with heart disease, providing real-time data to healthcare providers. By tracking heart rates, physical activity, and stress levels, these devices offer early warnings of potential complications, allowing prompt intervention.
The Future of AI in Healthcare
AI in healthcare is advancing rapidly, with emerging applications in areas like digital pathology, at-home diagnostics, and patient engagement. These tools enable remote care delivery, crucial for underserved populations and rural communities. Continued research, investment, and policy development will shape the future of AI in healthcare, promising a landscape of improved efficiency, accessibility, and patient outcomes.
FAQ
Q1: What are the main benefits of AI in healthcare?
A1: AI enhances diagnostic accuracy, enables personalized treatments, improves operational efficiency, and supports preventive care. By providing faster and more precise analyses, AI allows for earlier interventions and better health outcomes.
Q2: How is AI used in medical imaging?
A2: AI analyzes imaging data from X-rays, MRIs, and CT scans to detect abnormalities, often with a level of precision that matches or surpasses human specialists. AI in medical imaging accelerates diagnosis, improves accuracy, and reduces diagnostic errors.
Q3: What ethical concerns arise from using AI in healthcare?
A3: Ethical concerns include data privacy, algorithmic bias, and the risk of over-reliance on AI for medical decisions. Addressing these concerns requires robust security, diverse data training, and human oversight in AI applications.
Q4: How does AI contribute to precision medicine?
A4: AI analyzes genetic data and personal health metrics to create customized treatment plans, particularly valuable in cancer care. Precision medicine uses AI to predict how a patient will respond to specific treatments, reducing adverse effects and improving outcomes.
Q5: What are the limitations of AI in healthcare?
A5: Limitations include dependency on high-quality data, potential biases, and the complexity of interpreting AI models. Additionally, over-reliance on AI could undermine the critical role of healthcare professionals in medical decision-making.