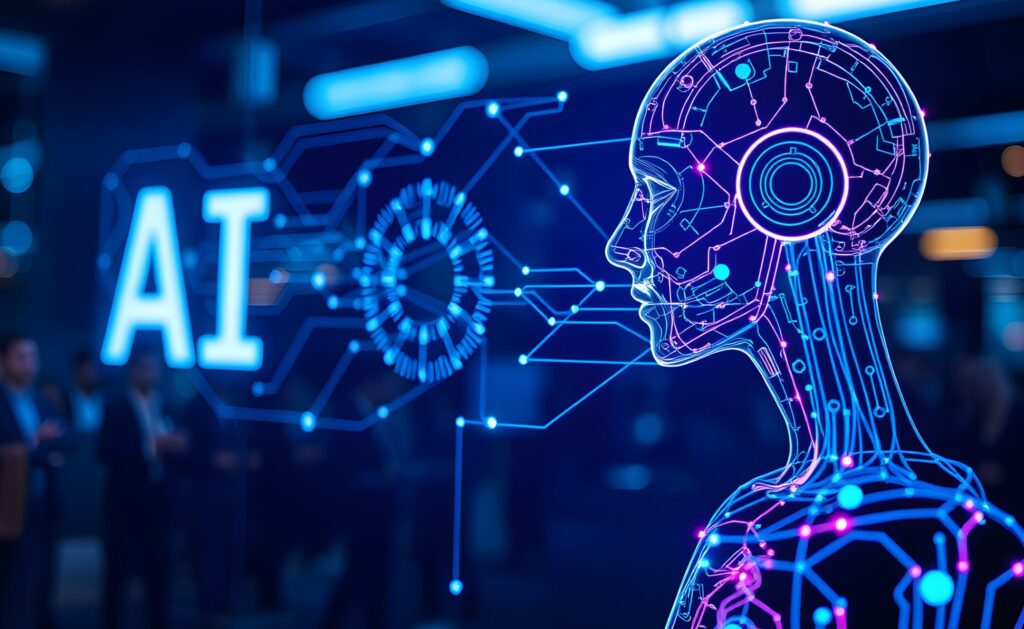
OpenAI’s o3 Suggests AI Models Are Scaling in New Ways — But So Are the Costs
Artificial intelligence (AI) has seen incredible advancements over the past decade, enabling innovations across every industry from healthcare to finance. OpenAI, a leader in the field, has been at the forefront of these developments. With the release of its most advanced AI model yet, o3, OpenAI is once again redefining the potential of machine learning.
o3 stands out for its ability to scale AI models in unprecedented ways, particularly in terms of reasoning. It leverages a technique known as “test-time scaling,” where additional computational resources are used during inference to solve more complex problems. This approach has led to significant improvements in AI’s ability to reason and handle difficult tasks. However, the remarkable advancements come with a downside: the increased computational resources required to operate o3 have sparked concerns about the model’s cost-effectiveness and its feasibility for widespread use.
This article explores the breakthroughs that o3 represents, its impressive performance benchmarks, and the rising costs associated with scaling such advanced AI models. By examining the trade-offs between innovation and sustainability, we will assess how OpenAI plans to address these challenges and what the future holds for AI at large.
The Evolution of AI Models
OpenAI’s journey from its early models to o3 is a story of rapid and consistent progress in the field of AI. The company’s first widely known model, GPT-2, showcased the potential of large-scale language models but was still limited by the computational constraints of the time. As these models grew in complexity, so too did the hardware required to run them, leading to innovations like GPT-3, which introduced significant improvements in natural language understanding and generation.
Following GPT-3, the introduction of GPT-4 pushed the boundaries even further, allowing for more nuanced and contextually aware text generation. However, despite these advancements, AI models were still somewhat constrained by their ability to handle more complex reasoning tasks.
This is where o3 comes in. OpenAI’s new o3 model is designed to address these gaps by using test-time scaling to adapt the model’s computational resources based on the complexity of the task at hand. This means that for more difficult problems, the model can utilize additional resources, dramatically improving its reasoning capabilities and overall performance. As a result, o3 can solve problems that its predecessors struggled with, opening up new possibilities in fields such as scientific research, healthcare diagnostics, and autonomous systems.
However, the very feature that makes o3 so powerful—the ability to scale dynamically—comes with a significant cost, which leads us to the next major issue with o3: its operational expenses.
Key Features of o3
o3’s innovation lies not just in its ability to generate text or recognize patterns but in its remarkable reasoning capabilities. At the core of these advancements is test-time scaling.
Test-Time Scaling
Unlike traditional AI models, which rely on static computational resources for both training and inference, o3 adapts its resource allocation dynamically during inference. When faced with a more complex problem, o3 can allocate additional computational resources, allowing it to perform significantly better on tasks that require deeper reasoning. This scalability is particularly useful in solving high-level problems that go beyond basic data analysis, such as medical diagnoses or legal reasoning.
Benchmark Performance
One of the most telling aspects of o3’s capabilities is its performance on the ARC-AGI benchmark. The ARC-AGI benchmark is a standard test for evaluating an AI model’s general reasoning ability, and o3 has achieved an astounding 88% score. This is a considerable improvement over previous models, such as o1, which scored only 32%. This leap in performance demonstrates o3’s ability to reason more effectively across a wide range of problem domains, making it a powerful tool for a variety of industries.
Practical Applications
The enhanced reasoning capabilities of o3 open up numerous potential applications. In healthcare, for example, o3 could be used to diagnose complex diseases or predict the outcomes of medical treatments with a higher degree of accuracy. In the financial industry, it could be used for advanced risk modeling, where the ability to think critically about financial scenarios is key. Additionally, o3’s ability to adapt to various industries means it could be used in customer service, legal consultations, and scientific research, among other fields.
The Cost of Innovation
While o3’s enhanced reasoning and scalability are impressive, they come at a high computational cost. The additional resources required for test-time scaling mean that running o3 is far more expensive than previous models.
Economic Implications
The most obvious consequence of these increased computational demands is the cost. Large AI models like o3 require powerful hardware and significant energy consumption to run. This translates to higher operational costs, which could make it difficult for smaller businesses or independent developers to access or utilize o3 effectively. Even larger companies may struggle to justify the high costs of running the model for certain applications, particularly when there are cheaper alternatives available for simpler tasks.
The expenses associated with operating o3 go beyond just the price of the hardware. The energy consumption required to power the model is considerable, raising concerns about the environmental impact of deploying such powerful AI systems at scale. This is a significant challenge for OpenAI, as it seeks to balance innovation with sustainability.
Industry Perspectives
Within the AI community, opinions on the cost of o3 are divided. Some experts argue that the breakthroughs made by o3 justify the higher costs, particularly in high-stakes industries where the benefits outweigh the expenses. Others, however, caution that the rapid increase in computational costs could become a barrier to widespread adoption. As AI models grow more powerful, the cost of using them may become prohibitive for many organizations, potentially stifling innovation.
OpenAI has acknowledged these concerns and is actively exploring ways to make o3 more affordable and accessible to a wider audience.
Strategies for Cost Management
To mitigate the financial burden associated with o3, OpenAI has taken several steps to optimize its use while still maintaining its performance.
Introduction of o3-Mini
In an effort to provide a more cost-effective alternative, OpenAI has introduced o3-mini. This version of the model is designed to offer many of the same reasoning capabilities as o3 but with a reduced computational footprint. By streamlining some of o3’s more resource-intensive processes, o3-mini allows businesses to take advantage of the model’s capabilities without the same high operational costs.
Optimizing Resources
OpenAI is also working closely with cloud service providers to optimize resource allocation. By leveraging the cloud’s scalability, OpenAI can ensure that o3 and o3-mini are used in the most efficient way possible, reducing the overall cost of running these models. Additionally, advances in hardware design and more efficient algorithms are helping to make the running of large AI models more sustainable from an energy perspective.
Competitive Landscape
o3 is not the only AI model aiming to redefine what is possible with reasoning and computational scaling. Competitors like Google are also working on their own advanced models, such as Gemini 2.0, which touts “flash thinking” capabilities that rival o3’s performance. These innovations contribute to the growing competitive pressure within the AI industry, where companies must balance the need for cutting-edge technology with the necessity of controlling costs.
Impact on Innovation
As companies race to develop increasingly powerful AI models, the cost of doing so continues to rise. While this competition drives rapid advancements in AI, it also raises questions about the sustainability of these innovations in the long term. OpenAI and its competitors will need to find ways to reduce costs while maintaining the high performance that makes their models so valuable.
Ethical and Sustainable AI
With the rising cost and resource demands of AI models like o3, ethical considerations have become a key part of the conversation.
Environmental Impact
The computational power required to run AI models like o3 is substantial, leading to a significant environmental footprint. OpenAI has stated its commitment to addressing this issue by exploring green computing solutions, such as more energy-efficient hardware and sustainable data centers.
Accessibility and Equity
Another ethical concern revolves around accessibility. The high costs of running models like o3 could limit access to only large corporations or wealthy institutions, potentially widening the gap between those who can afford to use advanced AI and those who cannot. OpenAI is aware of this issue and is working to ensure that the benefits of its AI advancements are available to a broader range of users.
The Future of AI Models
Looking ahead, the future of AI models will likely be defined by efforts to scale models like o3 while simultaneously addressing the rising costs and environmental concerns. OpenAI’s focus on optimizing resources and introducing models like o3-mini is a step in the right direction, but the industry as a whole will need to adopt sustainable practices to ensure that AI continues to evolve without negatively impacting society or the environment.
Predictions
AI models of the future will likely continue to scale in terms of both reasoning capabilities and computational resources. However, as the industry learns from the lessons of o3, there will likely be a stronger focus on creating models that are both powerful and cost-efficient, with sustainability playing a more prominent role in their development.
OpenAI’s o3 model represents a major leap forward in the field of AI, with its advanced reasoning capabilities and scalable design. However, the model’s reliance on significant computational resources raises concerns about its long-term feasibility and accessibility. As OpenAI works to optimize o3 and introduce cost-effective alternatives like o3-mini, the broader AI community will need to address the ethical and economic challenges associated with these powerful models.
The future of AI will depend on finding a balance between innovation, sustainability, and accessibility, ensuring that the benefits of these advancements can be shared by all.
FAQs
What is test-time scaling in AI?
Test-time scaling is a method that allows an AI model to dynamically allocate computational resources based on the complexity of the task it is working on. This technique helps the model handle more difficult problems by utilizing additional resources during inference.
Why is o3 considered so powerful?
o3 is powerful due to its advanced reasoning capabilities. By using test-time scaling, it can tackle complex problems more effectively than earlier AI models, making it suitable for applications in healthcare, finance, and other high-stakes industries.
What are the main challenges with o3?
The main challenges with o3 are its high computational and operational costs. The increased resource requirements make it expensive to run, which could limit its accessibility to smaller businesses and developers.
How is OpenAI addressing the cost issue with o3?
OpenAI is addressing the cost issue by introducing a smaller version of the model, o3-mini, which retains many of the same capabilities but with a lower computational footprint. Additionally, OpenAI is optimizing resources and working with cloud service providers to reduce the overall cost of running o3.
What is the environmental impact of models like o3?
The environmental impact of models like o3 is significant due to the large amount of computational power and energy required to run them. OpenAI is exploring energy-efficient hardware and sustainable data centers to mitigate this impact.
How will AI models evolve in the future?
AI models will likely continue to evolve in terms of reasoning capabilities and computational scalability. However, there will be an increasing focus on creating models that are both powerful and cost-effective, with sustainability being a key consideration.
Feel free to check out our other website at : https://synergypublish.com